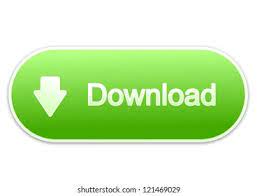
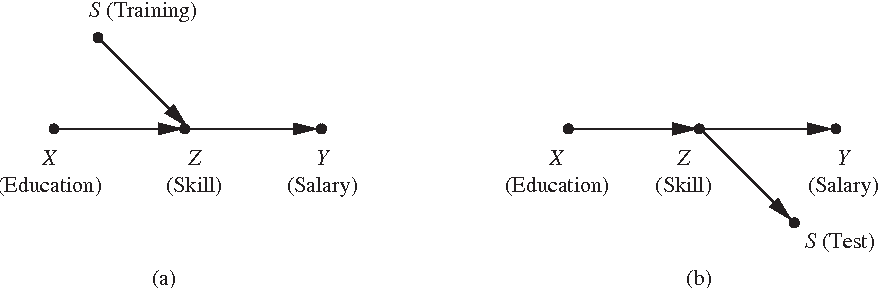
Pearl’s Causal Hierarchy and the Causal Hierarchy Theorem RL from a Causal Lens This module introduces some basic results in causal inference (SCMs and Graphs) and the 3-layer inferential hierarchy proposed by Pearl and collaborators.
#Pearl causality how to
"The deconstruction of paradoxes in epidemiology". Spurious causality Eating makes you faithful Will he cheat How to tell. "When Correlation Is Not Causation, But Something Much More Screwy". Make no mistake about it: this is an important book. Dr Judea Pearl has received the 2011 Rumelhart Prize for his leading research in Artificial Intelligence (AI) and systems from The Cognitive Science Society. New York City: Cambridge University Press. Causality will be of interest to students and professionals in a wide variety of fields. Causality: Models, Reasoning and Inference. These advances are illustrated using a general theory of causation based on the Structural Causal Model (SCM) described in Pearl (2000a), which subsumes and unifies other approaches to causation, and provides a coherent mathematical foundation for the analysis of causes and counterfactuals. It’s mini because of two reasons: first, it does not require the machine to understand natural language nor being able to communicate using it and, second, the story encode can do in any convenient way to pass the test, the machine does not need to encode it itself. The Mini Turing Test is a test defined by Pearl as a machine being able to answer causal questions about an encoded story, just as a human would be. And being able to imagine something that isn’t a fact is a crucial ability to causal reasoning, since it’s pretty much counterfactual estimation: you’re trying to find out how something would be if the facts would have been different.Īnd there’s more criticism about deep learning: it’d have succed, in fact, more in showing to us that some tasks we though were hard aren’t indeed then it has contributed to machine intelligence. He highlights that the world is not made of dry facts (the kind of thing associated in step 1), but they are glued up by causal-effect explanations. Alex Liu August 2005 This is a note on my reading Judea Pearl’s book Causality: Models, Reasoning, and Inference 1999 Cambridge University Press. It just associates a bunch of variables (\(X\)) to an outome (\(y\)), without any additional information. A Note On Causality: Models, Reasoning, and Inference by Judea Pearl By Dr.
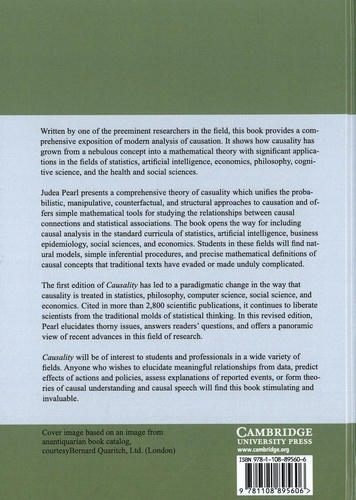
It is an exposition and analysis of causality.
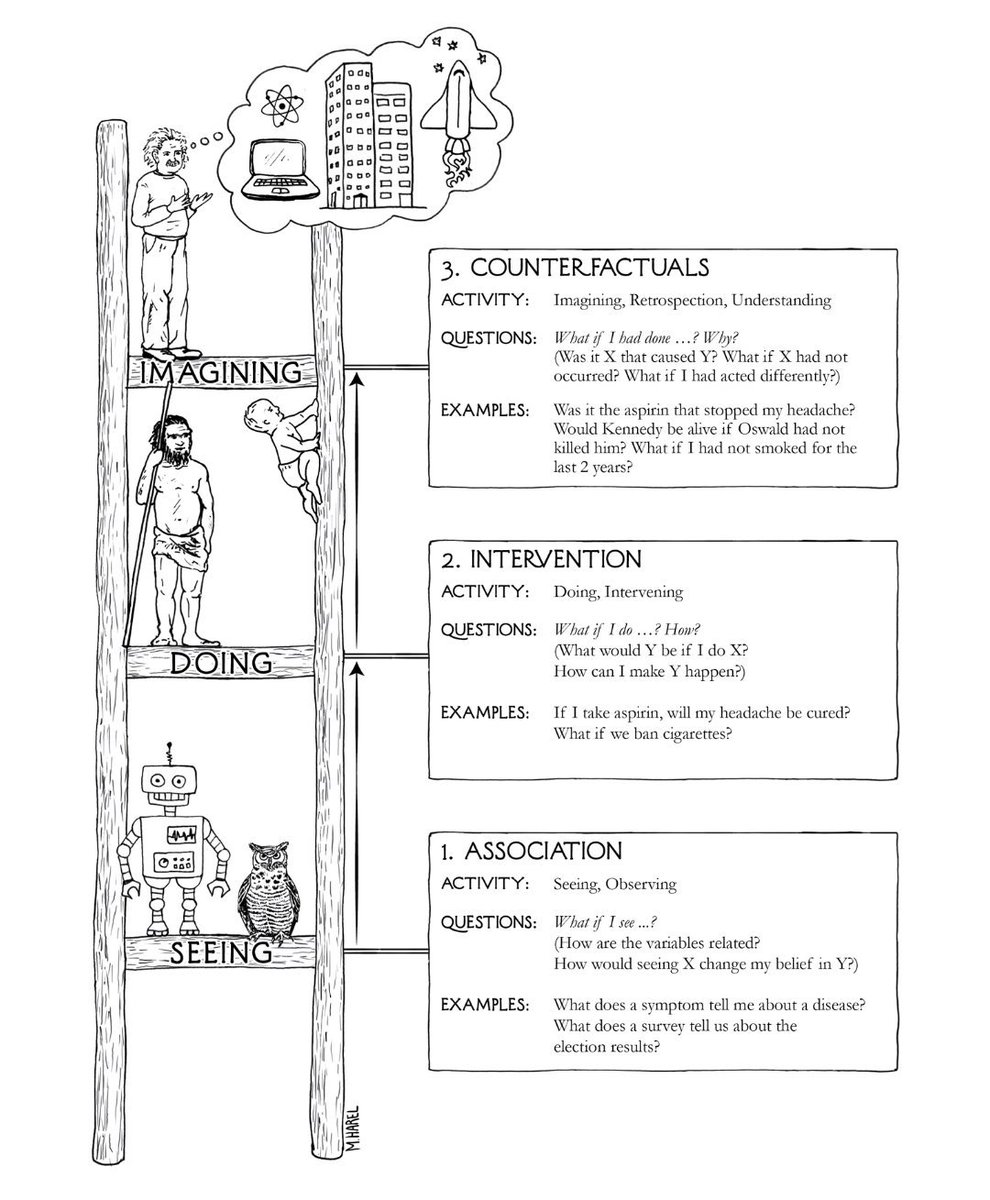

Causality: Models, Reasoning and Inference ( updated ) is a book by Judea Pearl. As you can imagine, traditional machine learning lies on the first one. Pearl asserts that causality has been mathematized10 although, as noted above, he adopts a more inclusive attitude towards causal claims than advocates of the RPOA in epidemiology. In Causality: Models, Reasoning, and Inference, Judea Pearl offers the methodological community a major statement on causal inquiry.
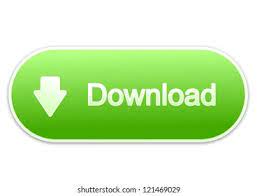